One big advantage of machine learning and artificial intelligence (AI) over more traditional means of analysis is that they can see things we cannot, such as hidden relations and correlations, among a myriad of other discoveries. On top of that, they can also do everything much more efficiently than us.
The advantages mentioned above have led to a wide range of machine learning and AI applications in recent years and in various fields such as business and retail. In fact, an AI-planned economy might not be that far-fetched of an idea based on how everything is developing these days. However, it is also seeing surging popularity in the healthcare sector.
Today, we are going to focus on one specific application of artificial intelligence in the healthcare sector, one that could save us time and potentially save more lives — radiotherapy. While we have yet to find a way to eradicate cancer once and for all, we might have found a better way to treat cancer through AI.
The Process of Radiation Therapy
You probably have a lot of questions about the use of AI in radiotherapy now — how does it even work? What exactly does AI have anything to do with radiotherapy? What do the machines analyze?
No worries, we will go into more detail in this article — bit by bit, little by little.
First of all, let’s try to understand the
target="_blank" aria-label="undefined (opens in a new tab)" rel="noreferrer noopener">process of radiation therapy and the steps involved. We can break the process down into seven sections:
- Imaging
- Treatment planning
- Simulation
- Radiotherapy accessories
- Radiation delivery
- Radiotherapy verification
- Patient monitoring
Imaging
Imaging is the first step in radiation therapy. Namely, this step involves diagnosing the patient for tumors, where other steps will then follow should the doctor discovers the presence of a tumor inside a patient’s body. During this step, the doctor estimates the tumor volume, shape, location, and surrounding organs that are at risk, alongside other important information.
At this stage, the doctor will also try to determine if there’s a distant metastasis — if not identified correctly, it can pose serious risks in the future.
Radiation therapy treatment planning and simulation
Radiation therapy planning and simulation can technically be grouped into the same step. It involves simulating the process by utilizing the information gathered (including tumor mass, height, weight, body mass index, previous exposure to radiation, and internal medical image of the patient) to calculate the outcome with different parameters in order to achieve the best results.
This is an extremely important yet complex step as it determines the risk and establishes the failure estimation and prediction. To successfully implement the therapy requires an extremely precise treatment setup, repeated monitoring, and understanding of the precise location of the target volume.
The treatment planning process can also be divided into three categories — knowledge-based, expert-based, or AI-based.
After the simulation phase, the doctor can then start the treatment planning and the dose calculation.
Radiotherapy accessories
Radiotherapy accessories is a step where the patient is immobilized with their movements restricted in order to have a more accurate estimation of the target volume location. It is usually carried out in the simulation stage and can be seen as a small sub-step in the whole process.
If you’re interested, there is research about the best clinical immobilization techniques for a precise and accurate dosage delivery.
Radiation delivery
Radiation delivery is the stage where a predetermined dosage of radiation is delivered to the patient, and it can be seen as the climax of the whole radiation therapy process. After all the preparatory work, ionizing radiation is delivered to kill the tumor cells while sparing or minimizing the energy deposition to healthy cells as much as possible.
There are also different radiotherapy modalities available now, such as stereotactic body radiotherapy (SBRT), volumetric modulated arc therapy (VMAT), Intensity-modulated radiation therapy (IMRT), proton therapy, electron therapy, and brachytherapy, each offering their own advantages and disadvantages in terms of speed and precision. We are not going to go into details about the modalities listed above, but we are sure you can find plenty of information on the internet.
Radiotherapy verification
Delivering radiation to human bodies is a serious affair. Therefore multiple precautions are in place to ensure the correct dosage and location of the radiation delivery, thus the need for radiotherapy treatment verification arises.
Dosimetric verification or a geometric localization would take place during the treatment. According to research, the process involves “measuring the dose at some points inside (natural cavities) or outside the patient, and comparing it to the dose at the same points calculated and predicted by the treatment planning system.”
See this as a quality assurance program where experts will ensure that the therapy is carried out correctly and precisely as planned.
Patient monitoring
The monitoring process takes place during the therapy and also after. During the therapy, the radiation oncologist would constantly monitor the patient and their daily treatment and would alter the radiation dosage based on those observations.
After the treatment, an observation period would ensue. It can last from months to years to ensure the therapy's success — that all the cancer cells have been eradicated and the patient is not at imminent risk of metastasis.
Personnel Involved in Radiotherapy
Now that you know the process, here is a list of personnel involved in the radiation therapy process:
Radiation Oncologists — physicians who oversee the radiation therapy treatment. They develop and prescribe the treatment, and they work with other members of the team to ensure the correct treatment delivery.
Medical Physicists — work directly with radiation oncologists to make sure everything, such as the dosage delivery, is done correctly; they also develop quality assurance programs.
Dosimetrists — as their title implies, these experts calculate the dose of radiation required to kill the cancer cells while sparing the healthy ones.
Radiation Therapists — work with radiation oncologists to carry out the treatments under their supervision.
Now that you have a better understanding of how radiotherapy works, we can finally take a look at AI radiotherapy implementations.
Artificial Intelligence in Radiotherapy/AI in Radiation Oncology
As we mentioned above, imaging is normally the first step in radiation oncology treatment planning — before we can cure cancer, we have to locate the cancerous cells first.
Based on the types of cancer detected, different imaging techniques are used to locate cancerous cells — computed tomography (CT), magnetic resonance imaging (MRI), and X-ray … just to name a few. As an example, let’s take a look at how AI and machine learning can help correctly identify breast cancer during the imaging stage.
Using AI in breast cancer imaging
Ultrasound is normally used to detect breast cancer in women. After obtaining the ultrasound images, they should then be segmented into functional tissues in order to locate the tumor and determine the course of treatment required.
However, manually segmenting the images is largely reliant on the skill and experience of the personnel in charge, which is prone to human error. On top of that, it is also extremely time-consuming for radiologists to review hundreds upon hundreds of images.
This is why the use of convolutional neural networks (CNNs) was explored in one research — with promising results. Using CNNs, the team was able to segment breast ultrasound images into four major tissues: skin, fibroglandular tissue, mass, and fatty tissue, with an 80% overall accuracy; another metric used, known as the Jaccard similarity index (JSI), yields an 85.1% value, which outperformed their previous study.
Another imaging technique used in identifying breast tumors is mammograms. In recent years, computer-aided design (CAD) programs have been used to improve the efficiency in analyzing the images — again, imagine how much time it’d take to review the images manually. Another research also utilized CNNs and fed the network with around 45,000 images as their trained dataset and compared its performance with the CADs currently in use.
The results? It outperforms the traditional CAD system at low sensitivity and performs comparably at high sensitivity. Meanwhile, no significant differences were observed compared to certified screening radiologists on a patch level.
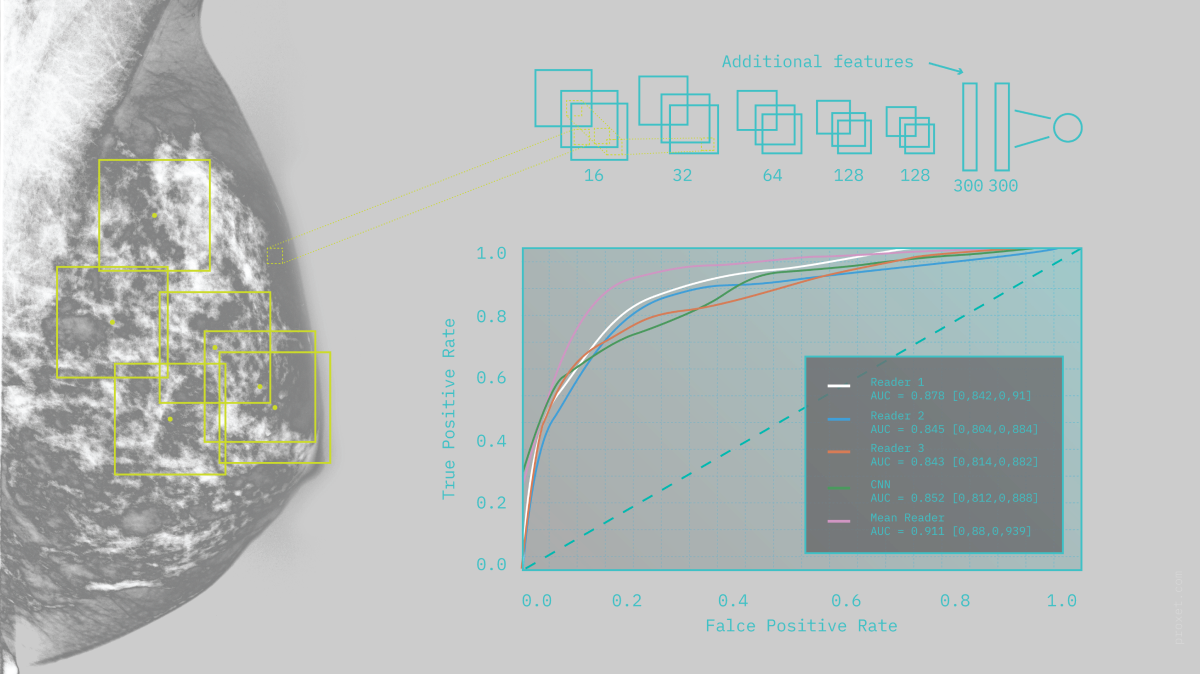
Exciting, isn’t it? Considering breast cancer alone contributed to 685,000 deaths globally in 2020.
Automated treatment planning in radiotherapy
Automated treatment planning is another use of AI in radiation therapy that is currently being explored. As we mentioned above, numerous parameters and variables are involved in the planning process to ensure the best outcome.
To simplify the calculations and thus, the planning process, K-nearest neighbors (KNNs) have been used in CAD systems in one research, aiding the oncologists by matching the current dataset to the most similar training data. The plans were then further fine-tuned using stochastic optimization.
The automated treatment plan offers similar performance to those conducted manually while significantly reducing the time to less than a minute.
AI-aided radiotherapy quality assurance
Lastly, let’s take a look at how AI can ensure the safety of radiation therapy.
Dr. Qiongge Li from Johns Hopkins University School of Medicine developed an anomaly detection algorithm to ensure therapy safety.
"It is important to address the detection of prescription errors in radiotherapy, even if this is a rare event."— Dr. Qiongge Li, Medical Physics Resident at Johns Hopkins University Hospital.
Traditionally, quality assurance in radiotherapy relies on peer-reviews that, despite the best efforts, cannot account for every single human error — in fact, in one specific study, only 67% of the prescription errors were detected in a peer-review chart with inserted simulated plan anomalies.
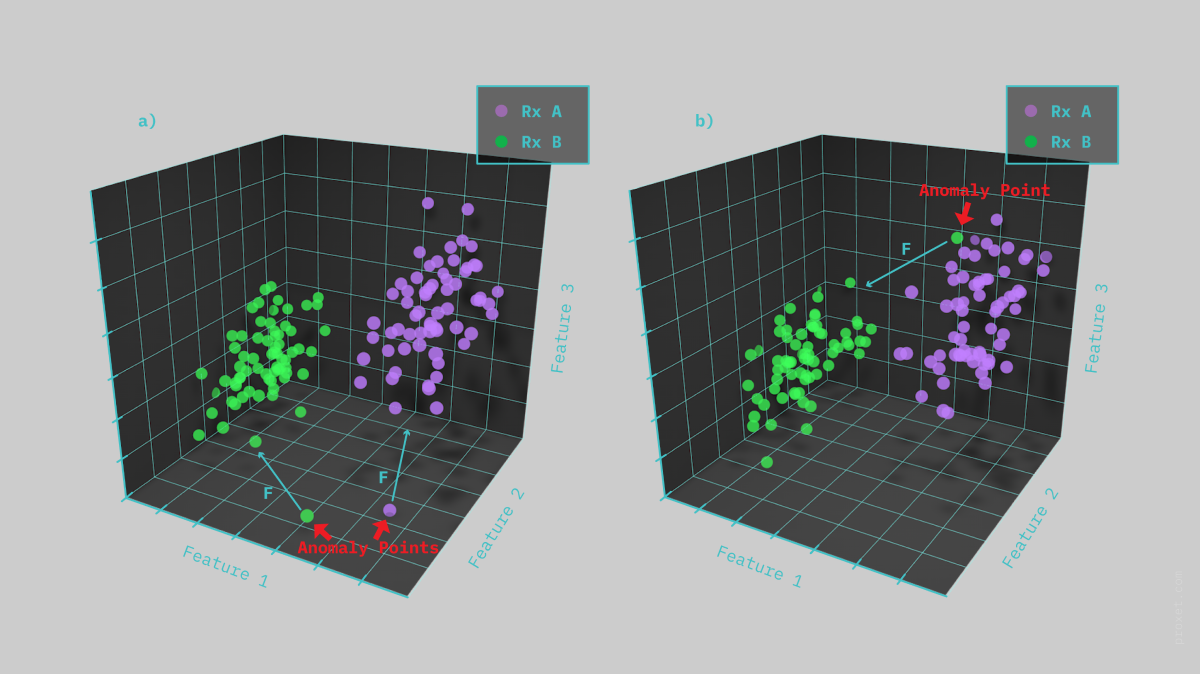
Using data from historical databases, Dr. Li and her team developed an algorithm that detects anomalies in the prescription. They were able to do so by using mean feature distances between all patient pairs in the historical database. They fed 11,062 historical thoracic cancer treatment plans to the system as their trained dataset, and it outperformed all three doctors in terms of recall, precision, F1, and accuracy. On top of that, it also took one second to run the model as opposed to the 15-30 minutes that it normally takes.
"Developing a fully automatic and data-driven tool for assisting peer-review chart rounds and providing that extra safety to our patients is in great need."— Dr. Qiongge Li, Medical Physics Resident at Johns Hopkins University Hospital.
The Future of Radiotherapy
"Artificial Intelligence (AI) ensures that cancer care can be more accessible, accurate, and improve the speed of diagnosis, aid clinical decision-making, and lead to better medical outcomes."
— Ashok Kakkar, senior managing director at Varian India.
With the rapid development and growing application of AI, it is believed that the technology will transform radiotherapy. While the technology might not be able to fully take over the field as it still requires a certain degree of expert involvement in diagnosing and treating cancer, AI could facilitate a faster and more accurate diagnosis through automation.
The use of AI, alongside the breakthrough of different innovative developments such as 4D imaging and
target="_blank" aria-label="undefined (opens in a new tab)" rel="noreferrer noopener">nanotechnology, is expected to accelerate the global radiotherapy market growth. According to
target="_blank" aria-label="undefined (opens in a new tab)" rel="noreferrer noopener">research, the market is expected to grow at a compound annual growth rate (CAGR) of 8.4% between 2020 and 2030.
"We have seen the widespread use of AI in the healthcare sector with promising results. Radiotherapy is only one of the many fields that would embrace the power of AI in the coming years."
— George Serebrennikov, COO at Proxet (ex - Rails Reactor) – a custom software development services company.
While the future of radiation oncology might not be fully reliant on AI, we have a firm belief that AI will be an integral part of its development.
The use of AI exists across different sectors, and Proxet, as a provider of software development services, has ample experience in utilizing AI and machine learning to provide trustworthy and reliable IT solutions. Are you wondering how your business, too, can embrace the versatility of AI? Contact us for more information.