Big data has arrived for healthcare and changed the way it works. Technology has improved medicine’s operational and financial efficiency and revolutionized clinical analytics. Healthcare big data analytics is growing rapidly according to Inkwood Research, with the market projected to grow 19.39% annually, reaching $97 billion by 2027. Several factors drive this large, steady growth:
- increased implementation of IoT and healthcare wearables
- overall advancement of the technological sector
- deployment of cloud technologies
- government initiatives promoting use of big data
The business community has come to see big data analytics as a value-driver and business component in its own right. Healthcare providers partner with software developers and digital companies to weave data into operations and derive value. And big data has gone from being a nice perk to a competitive necessity. Additionally, this pandemic has formed a new normal where a company’s ability to adapt determines whether it survives and thrives, or fails.
These days, healthcare businesses must be data-driven, predictive, proactive, transparent, accurate, deeply personalized, responsive, etc. Given the multiple sources of data and their high volume, a unified analytical tool must be in place to integrate informed decision-making, superior customer service, and administration.
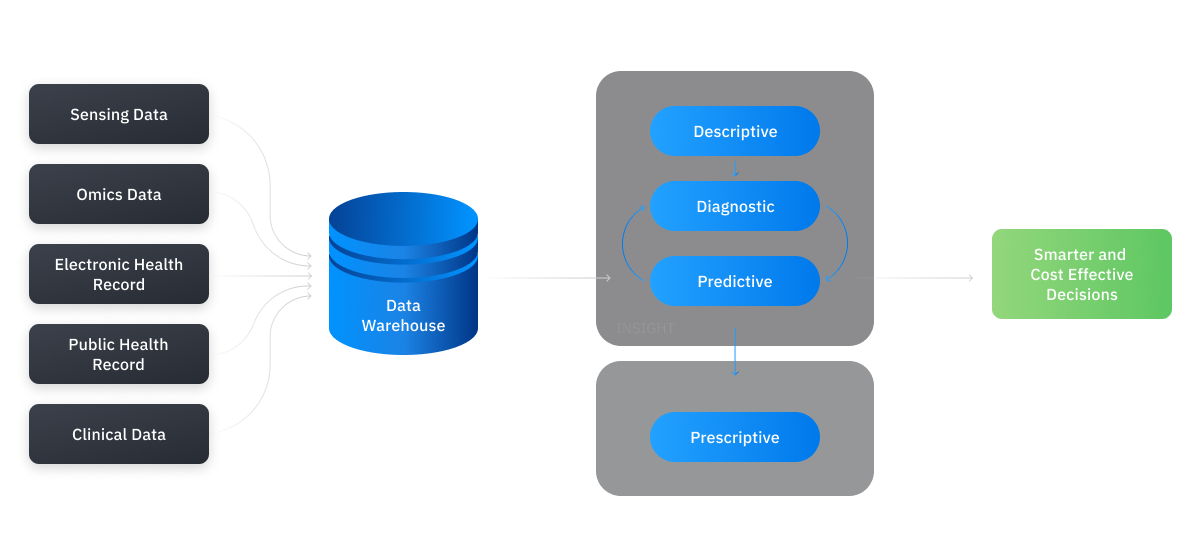
“A data scientist is someone who can obtain, scrub, explore, model, and interpret data, blending hacking, statistics, and machine learning. Data scientists not only are adept at working with data, but appreciate data itself as a first-class product.”
— Hillary Mason, Founder, Fast Forward Labs
Analysis and Classification of Data
Data creates no value by itself. It drives results when a chain of operations is performed. It starts with data mining, which is used to develop analytical models to analyze raw data on symptoms and make predictions about diseases. Individual’s medical files can be redundant, incomplete, and inconsistent, but predictions are needed immediately. Fortunately, data mining involves pre-processing, analysis of patterns, and use of mining algorithms to make predictions quickly.
Data mining both provides a big picture and looks at the available information from different angles to drive insights. Data classification is a process of generalizing known structures to be applied to new data. Analytics apply the following classification techniques:
Decision trees
The structure has root nodes, branches and leaf nodes. This technique is self-explanatory and easy.
Naive Bayes Classifiers
This type is based on Bayes’ theorem where assumptions between features are characterized by strong independence. The Bayes classifier is used to calculate the probability for every specific feature.
Artificial Neural Networks
ANNs work on the basis of connected artificial neurons. The main benefit of this classification technique is that it can work with incomplete data.
Each classification technique can be enhanced by raw data pre-processing and combining feature selection methods. Classification accuracy increases with the reduction of attribute numbers.
Individuals and Variables of Data
To understand the basics of how data is sorted out, let’s dive a little deeper into the field of statistics. In medical data sets, an individual is captured in the dataset as an object. Variables present the characteristics of an individual. Let’s look at an example:
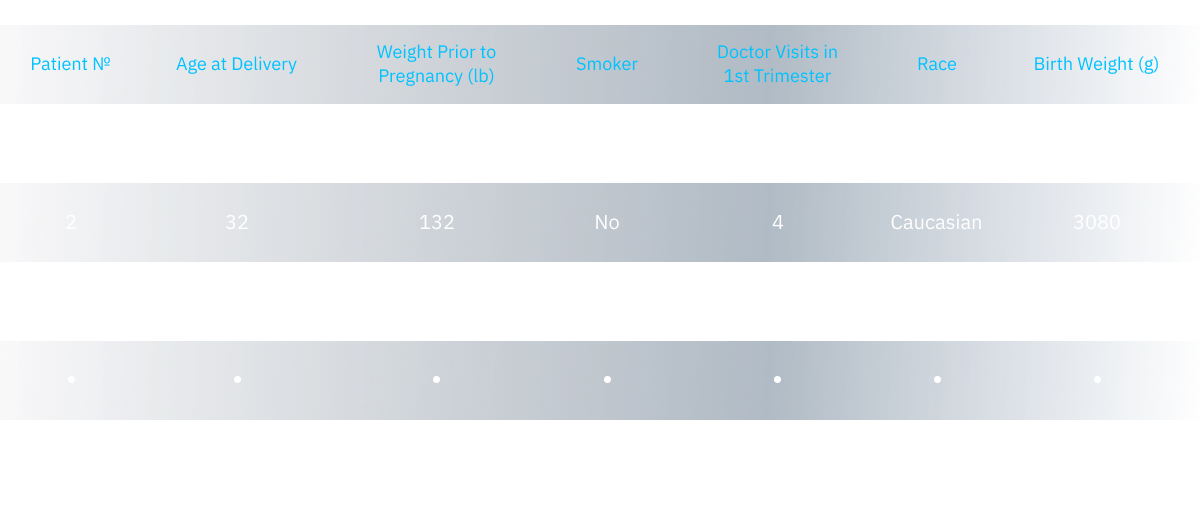
Medical Records Dataset Example by Lumen
In this medical survey, the researchers needed to identify variables connected with low birth weights. Patients (mothers) are the individuals, and all the other columns, from “age” to “birth weight,” are variables.
Variables fall into two types: categorical/qualitative and quantitative. Categorical variables take label values. In the example above, “smoker” is a categorical variable as a mother can either be a smoker or non-smoker. Quantitative variables, on the other hand, imply numerical values. In this table, “age,” “weight,” and “height” are quantitative.
Data Usage. Who Benefits at the End of the Day?
Big data analytics has impacted every stakeholder in the healthcare industry. Big data addresses issues that were previously unsolvable by conventional software or analytic methods. Data analytics optimizes workflows, enhances the results of clinical trials, boosts the productivity of medical organizations, and makes healthcare professionals more effective. Ultimately, analytics increases the revenues of a healthcare facility while controlling the costs.
Predictive analytics can significantly improve personal medicine. Doctors and disease researchers can find previously unknown cures. Data analytics provides a personalized approach, taking into consideration the unique genome of each patient. Previously, doctors could not access the complex data sets behind genomes. Now, big data can analyze the genome’s huge number of features and characteristics to find new correlations, uncover new patterns, and make predictions.
Big data analytics perfectly addresses the rise of high-cost patients and high-risk patient cases. According to Health Affairs, around 5% of US patients account for 50% of American healthcare spending. The resources at medical organizations are increasingly stretched thin. Big data can identify high-cost patients based on behavioral patterns and socio-economic factors, and use algorithms to reallocate resources and deploy them rationally.
Big data analytics delivers patient predictions for improved staffing. Total healthcare spending and the total number of patients needing treatment are growing. Staff currently accounts for roughly 50% of hospital operating costs. Data used for staffing is based on historical trends and contains inaccuracies, which leads to overstaffing and understaffing, which are equally bad for the medical business. Predictive analytics can improve staffing by accurately estimating admission rates, real patient demand, and point-of-care requirements for a given time frame.
Big data plays a crucial role in real-time alerting, analyzing patient data instantaneously to provide doctors with action plans. It is the next level of healthcare delivery: wearables accumulate data on the patient, and puts it in the cloud or large database storage. This requires online tools to monitor these immense data streams and make timely and correct recommendations.
“We want to use AI to augment the abilities of You have these healthcare systems who are basically sticking to a portal and they’re kind of looking to their health IT vendors—who they’re already paying lots of money—to roll out mobile apps, telemonitoring solutions, and things like that. And the vendors are like, 'The doctors and the hospitals they tend to want things to get them the meaningful use dollars.' So it’s like, who’s going to move first to these newer technologies?people, to enable us to accomplish more and to allow us to spend more time on our creative endeavors.”
— Naveen Rao, Analyst, Chilmark Research
Big Data Examples in Healthcare
There are many tools being successfully applied in healthcare around the world and bringing tangible benefits to medical institutions. Here are some of them:
MapR Converged Data Platform
Valence Health used MapR software to build a data lake on the basis of several thousand data feeds with 45 types of data. The data feeds include everything from lab tests results to claims and payments. It takes about 20 minutes to process this data.
UnitedHealthcare
UnitedHealthcare collaborates with 85,000 doctors and over 6,000 hospitals serving 51 million people. Through their health insurance platform, claims are paid on time and correctly. A predictive analytics algorithm continually monitors the transactions stream for inaccurate claims and fraud.
In an environment of growing demand, big data solutions are exploding. Proxet has been working in the healthcare industry for years, accumulating experience and finding innovative solutions for each company.
“Working with healthcare insurance, we do our best to approach data stream processing from multiple angles so our clients can drive value from their data and make informed decisions based on analytics. Predictive analytics, data science, ML and AI algorithms have been our friends for a long time. We know how to unlock the tremendous potential of each one so that the businesses we work with see immediate effects.”
— Vlad Medvedovsky, Founder and Chief Executive Officer at Proxet, a custom software development solutions company, expert in bringing transformation to health insurance businesses.